7 things you need to know about FaceReader
Are you looking for guidance in getting started with your facial expression analysis? Or want to get more out of your FaceReader setup? Keep reading to learn 7 essential things you need to know about FaceReader!
Posted by
Published on
Tue 15 Apr. 2025
Topics
| FaceReader | Facial Expression Analysis | FACS | Measure Emotions |
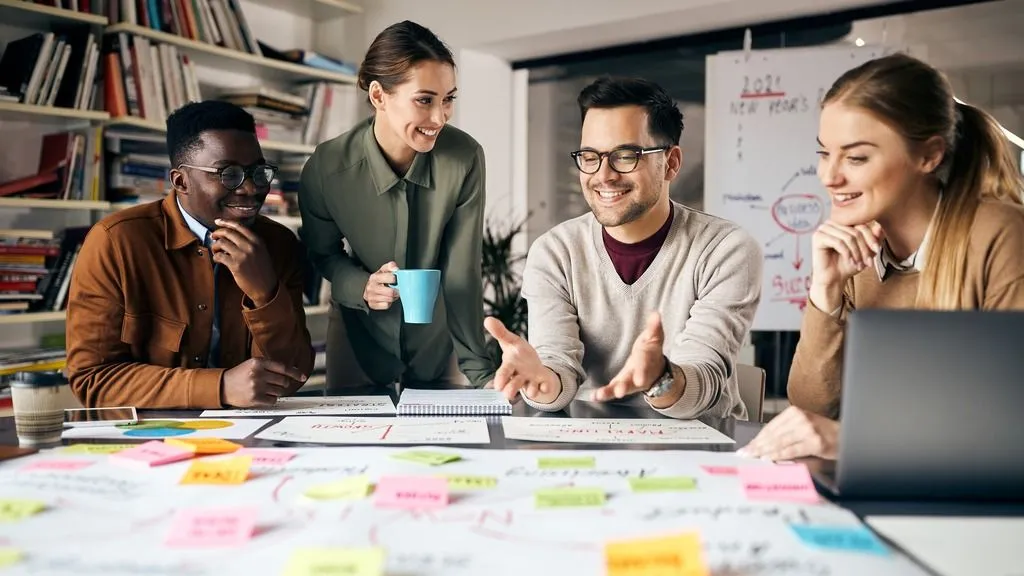
We received a wide range of questions during a live Q&A session for FaceReader users with NoldusCare—from basic how-to's to detailed questions about methodology and validation. In this blog post, we'll go over 7 of the questions people asked about FaceReader.
1. How does FaceReader calculate and classify facial expressions?
The FaceReader algorithm follows a three-step process. First, it detects the face in an image. Then it builds a face model based on the 468 facial landmarks in FaceReader. Finally, it uses a trained artificial neural network (based on 100,000 images) to classify emotional expressions and facial states.
The expressions classified by default include the six basic facial expressions—happy, sad, angry, scared, surprised, and disgusted—plus contempt and a neutral state. Additional modules allow you to measure Action Units, heart rate or heart rate variability, and even consumption behavior like chewing or intake.
You can monitor these over time and visualize the intensity of each expression and Action Unit on a timeline or in a line chart.
2. How is the Action Unit module validated, and how accurate is it?
We validated the Action Unit module in FaceReader using the Amsterdam Dynamic Facial Expression Set (ADFES), a dataset with 22 individuals displaying nine emotional expressions. These expressions were manually coded by certified FACS coders, and FaceReader’s outputs were then compared to those human ratings.
The agreement between the two FACS coders was 0.83, and the agreement between FaceReader and the human annotations was 0.81. This high level of agreement indicates strong validity, especially considering how complex facial coding can be.
| Read more on how FaceReader is validated.
FREE WHITE PAPER: FaceReader methodology
Download the free FaceReader methodology note to learn more about facial expression analysis theory.
- How FaceReader works
- More about the calibration
- Insight in quality of analysis & output
3. What are best practices for camera positioning and lighting during recordings?
Camera placement and lighting are key factors for ensuring high-quality data. FaceReader works best when the face is fully visible, evenly lit, and facing the camera.
A webcam placed right above the screen is typically the best option—it gives a frontal view without being too intrusive. Placing the camera too low (for example, at table level) can lead to false readings. For example, inflated eyebrow positions may look like surprise.
Lighting is equally important. You want diffuse lighting from the front to avoid strong shadows. Daylight works well, or you can use desk lamps or photo-type lighting with soft filters. Avoid backlighting, as that can darken the face. You should also avoid harsh overhead lighting since it can create shadows under the eyes or nose that interfere with your analysis.
| Get more tips on how to optimize your FaceReader setup.
4. What is a dominant expression in FaceReader?
A dominant expression in FaceReader is simply the expression with the highest intensity at a given moment. For example, if someone is smiling, but also raising their eyebrows slightly (a mix of happy and surprise), the dominant expression might still be coded as happy if its intensity is highest.
Even when an expression isn’t dominant, FaceReader logs the intensity of all expressions frame by frame. That means you can still analyze less prominent responses or micro-expressions—like surprise during a happy moment—by exporting the data from the expression line chart.
5. How can I compare responses between stimuli or between participants?
You can use the Project Analysis module for both within-subject and between-subject comparisons. For example, if participants watched two different videos, you can use the “Compare” function to visualize average responses—such as mean happiness or valence—across those stimuli. Keep in mind that for meaningful interpretation of FaceReader results, you always need to consider the context in which certain facial expressions were recorded.
You can also compare individuals by selecting participants manually. For example, you might want to see how participant A and B responded to the same video. FaceReader includes t-tests to help identify these differences.
6. What is a good workflow for batch processing a large number of videos?
If you're dealing with large datasets, batch processing of pre-recorded videos in FaceReader can save a lot of time. This means using the Project Wizard to load multiple videos automatically. Instead of manually adding each participant and assigning their video, you can select a folder and FaceReader will create one participant per video.
Once everything is loaded, the batch analysis feature will process each video one by one. To save time, you can also adjust the sample rate to analyze every third frame instead of every frame. For videos recorded at 30 frames per second, this means FaceReader analyzes 10 frames per second, which is still well above the 5 frames-per-second minimum needed for reliable results—but done much faster.
It’s worth noting that FaceReader does not autosave. So after a batch run, make sure to save the project to avoid losing data. Look for the asterisk (*) next to the project name—that means you’ve made changes that haven’t been saved yet.
7. Can I create my own custom expressions in FaceReader?
Yes! In FaceReader, you can define custom expressions based on Action Units, head movements, or other facial states.
You can build expressions using logical operators and thresholds. It's also possible to simulate the effect of different Action Unit intensities to test how your custom expression works. This is especially useful if you want to detect states that aren’t in the default set, like embarrassment, boredom, or pain.
Keep in mind that creating meaningful custom expressions requires research. Start by observing your participants in the context where that emotion or state is expected to occur. Then experiment with building expressions from the Action Units and visual features that match your observations.
RESOURCES: Read more about FaceReader
Find out how FaceReader is used in a wide range of studies and how it can elevate your research!
- Free white papers
- Customer success stories
- Featured blog posts
Facereader: a powerful tool for your research
This blog posts highlights just how versatile FaceReader is. Whether you’re running a simple usability test or building a custom model to detect subtle facial expressions, there’s a lot you can do with the software.
At the same time, good results depend on the right methodology: clear lighting, thoughtful design, and a strong understanding of what FaceReader is measuring.
Your research deserves the best
Got a question that wasn’t covered? Feel free to contact us! You can also find more in-depth information on the FaceReader resources page.
Or join our live Q&A sessions—exclusively available to NoldusCare members—where you can ask the experts about best practices.
Related Posts
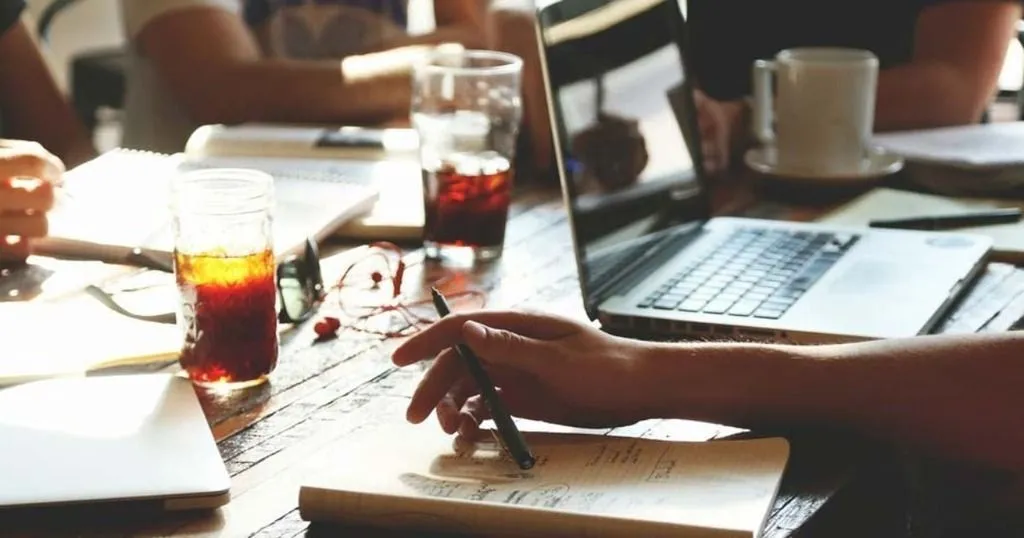
Facial expression analysis in video content marketing
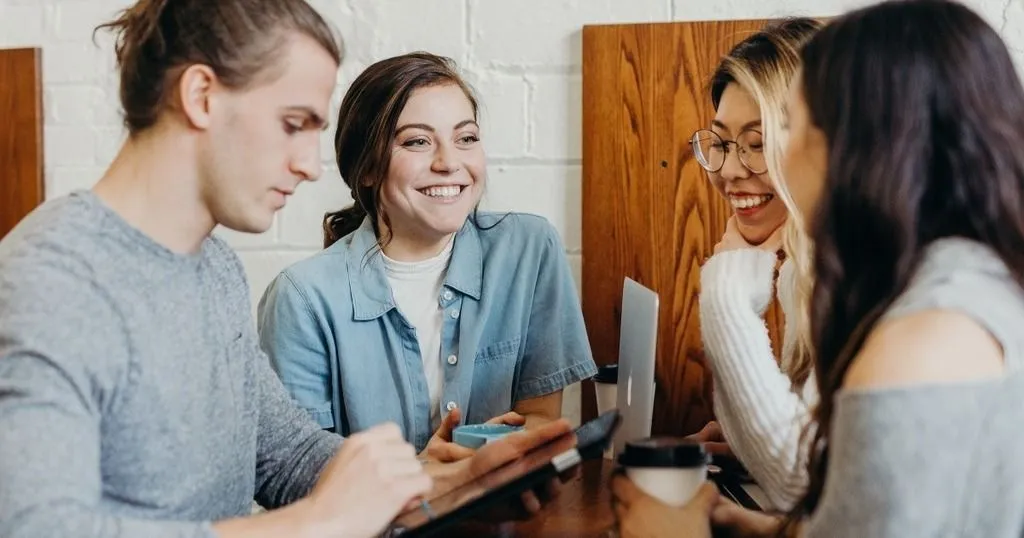
How to measure emotions
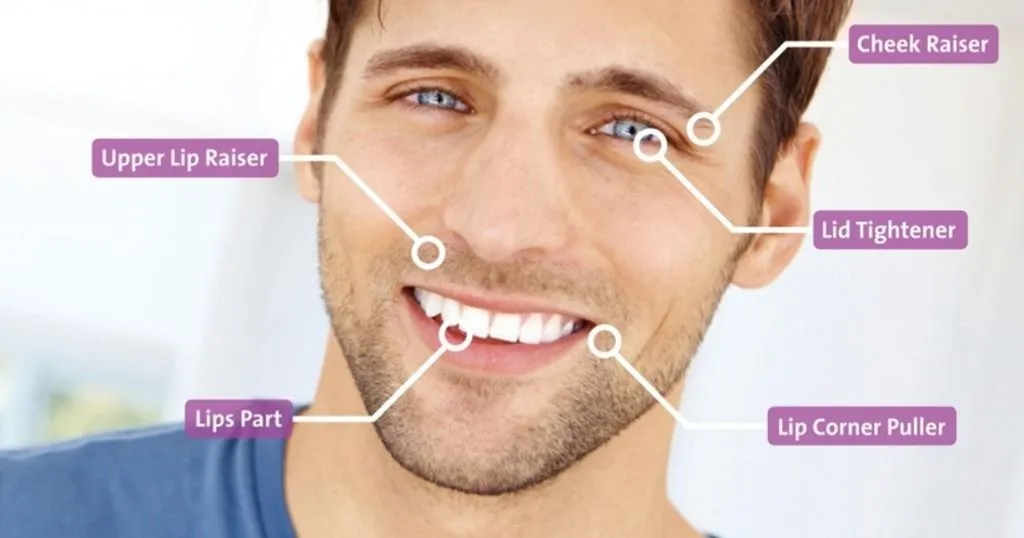