Facial expression analysis in video content marketing
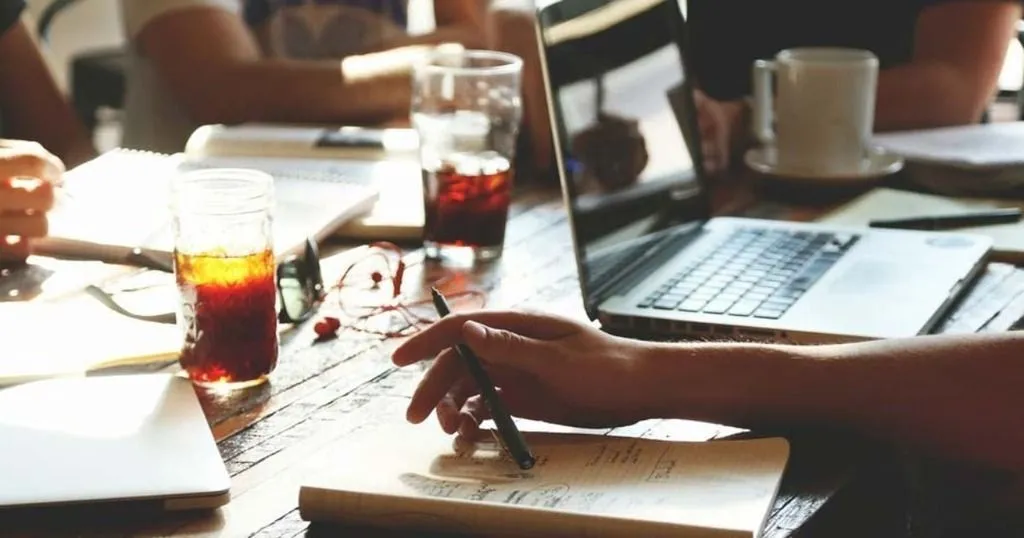
This study shows that emotions in company messages or advertisements could be used to build and measure brand value. This measure can thus be added to social media metrics guidelines to assess success rate.
Posted by
Published on
Thu 22 Feb. 2018
What if you could predict the popularity of campaign videos? Corporate communications can incorporate neuromarketing and nonverbal communication analysis as social media metrics in order to understand not only what content is effective, but also how it should be presented.
Campaign videos are often distributed via a video platform such as YouTube. The more views they get, the more successful the campaign becomes.
Automatic facial coding
What video will be successful? The study presented here concludes that it is not only the content that matters, it is also the body language -- in this case the facial expressions -- that influence the success rate of a video campaign. In this study, Amsterdam based researchers used two measures to assess behavior: automatic facial coding and video views on YouTube.
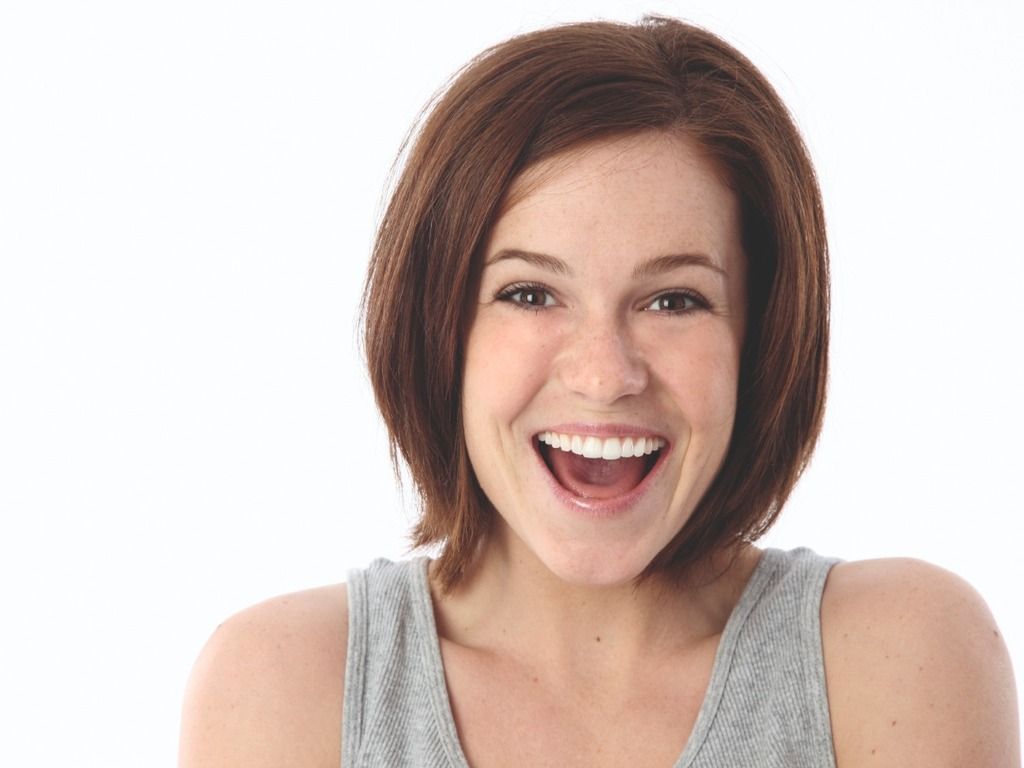
Measure success
This proof-of-concept study shows that the emotional face behavior of actors in company messages or advertisements could be used to build and measure brand value. This measure can thus be added to social media metrics guidelines to assess success rate.
Predict video popularity
Researcher Peter Lewinski from the University of Amsterdam looked at micro expressions of the face in great detail. In total, he analyzed 25,000 video frames of 16 speakers in order to predict video popularity over time. He presented his research in the Journal of Neuroscience, Psychology, and Economics.
FaceReader software
FaceReader software is used in this type of research, and is used worldwide at universities and companies to assess emotions automatically. It is research software that has a large installed base in different market segments.
By analyzing facial expressions using video images, you can get insight into 10,000 combinations of facial movements (Ekman & Rosenberg, 1997). The Active Appearance Model in FaceReader creates a three dimensional superimposed model of a face with over 500 hyper connected tracking points. FaceReader then uses a neural network trained on more than 10,000 facial images depicting basic emotions. It uses this network to recognize a particular expression.
Campaign video analysis
For this project, Lewinski selected sixteen ING Private Banking and ABN AMRO campaign videos, where the speaker was forward-facing, under good lighting conditions, and speaking to the YouTube channel watcher. All 16 campaign videos were analyzed using FaceReader software to get insight into the emotional level of communication of the ABN AMRO and ING speakers in the video.
FaceReader analysis showed that the lack of facial emotions and the presence of facial non-emotions were important indicators of a video’s popularity. The data shows that the fewer emotions that make you feel like you want to approach the person expressing the emotion (happy / sad), the more popular the video.
No emotions please
Concluding, the research outcomes show that in case of professional institutes such as these banks, a simple and non-emotional nonverbal message is what viewers and potential customers find most compelling. Happiness and sadness might just be too out of context in this case.
It is explained that social media users who watched the videos may have assumed the speakers to be employees of the bank, perhaps causing this serious tone of voice to be much appreciated.
Context matters
Lewinski mentions: “Several factors, for which we didn’t control, could have influenced the number of video views.” It is a challenge to predict success of a video, but Lewinski concludes that context certainly matters, like the bank employee (or actor) presenting in a simple and non-emotional nonverbal matter.
Reference
Lewinski, P. (2015). Don’t look blank, happy, or sad: Patterns of facial expressions of speakers in banks’ YouTube videos predict video’s popularity over time. Journal of Neuroscience, Psychology, and Economics, 8(4), 241-249. https://psycnet.apa.org/record/2015-47847-001
Related Posts
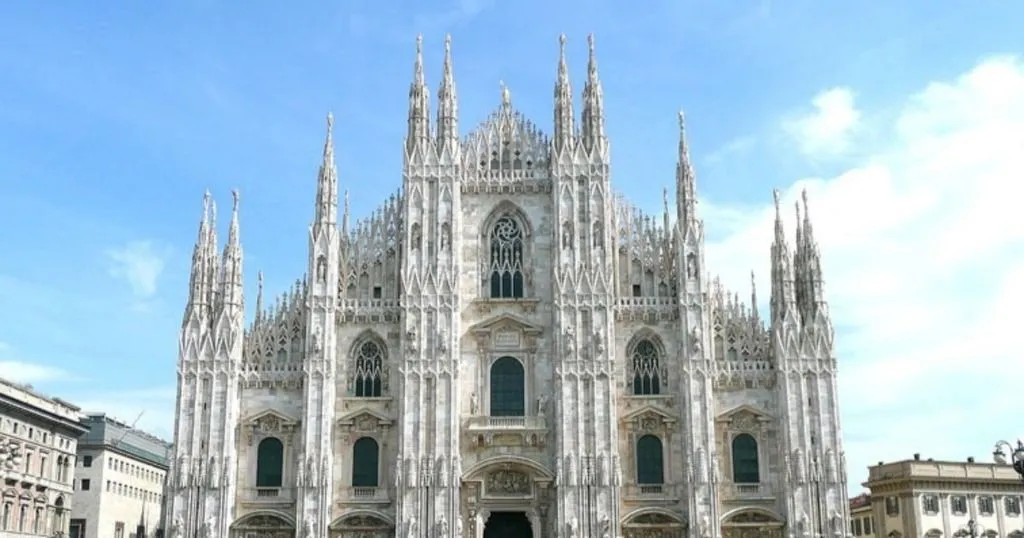
The man in the maze: A behavioral science journey into consumer studies
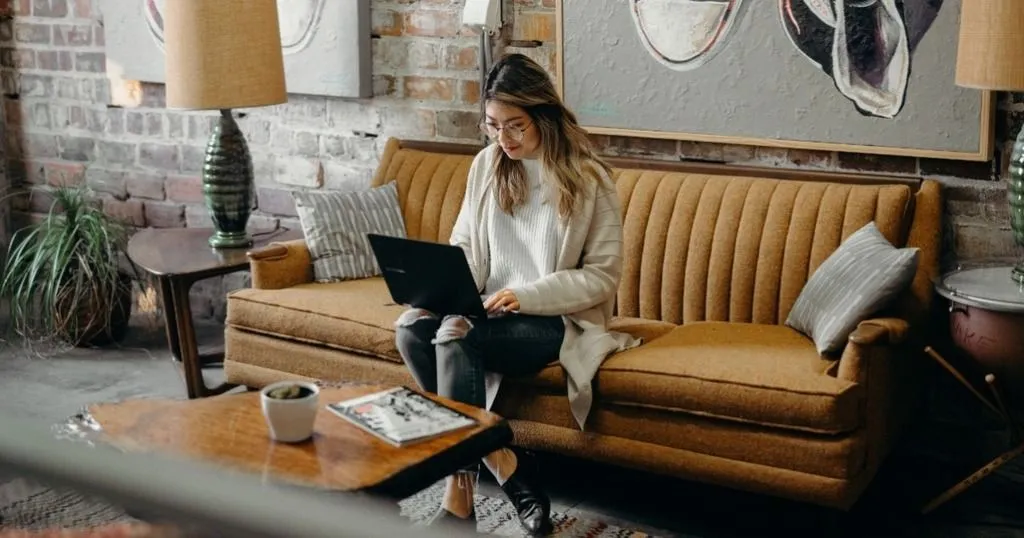
The influence of ad-evoked emotions on brand attitudes
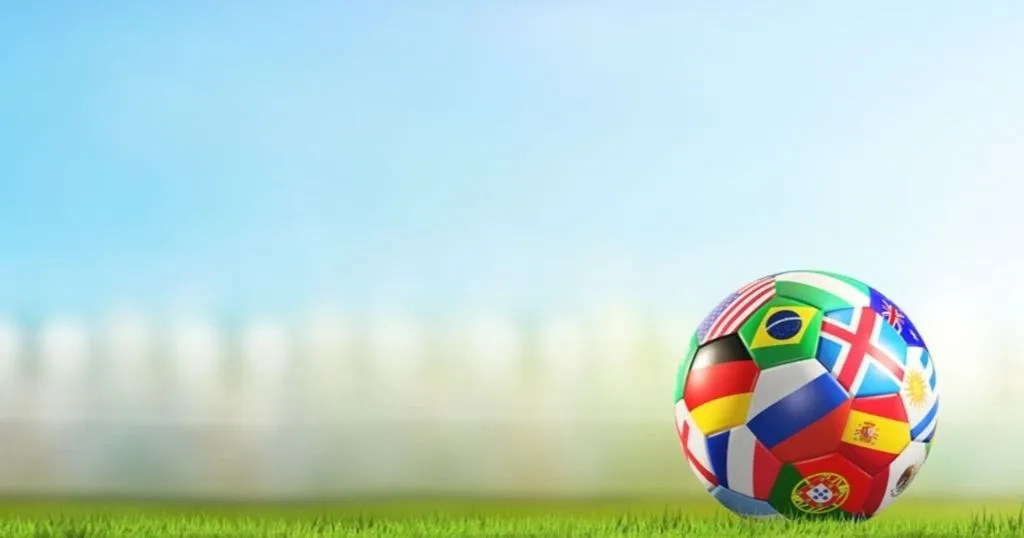