Creating a custom expression for engagement: a validation study with FaceReader
The concept engagement is gaining more and more attention. Many companies are looking for ways to increase consumer engagement. But, how do you know a consumer is feeling engaged?
Posted by
Published on
Mon 18 Jan. 2021
Topics
| Action Units | Consumer Behavior | Emotions | FaceReader | FaceReader Online | Measure Emotions |
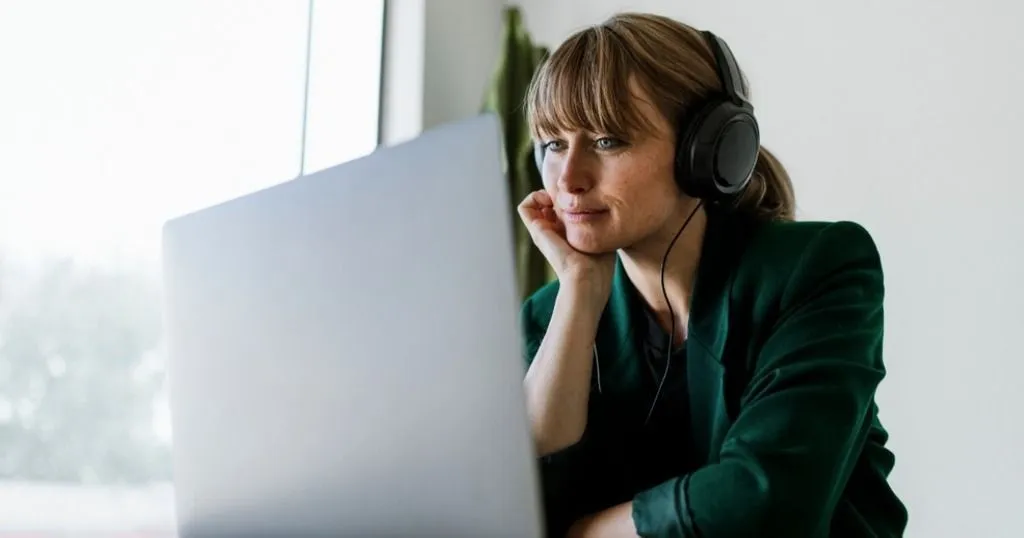
By Dayenne Sarkol-Teulings, graduate student at Applied Cognitive Psychology at the University of Utrecht, The Netherlands.
During my 3-month graduate research internship project for my master Applied Cognitive Psychology at the University of Utrecht, I was asked to create a custom expression for the emotional state engagement. In this blog, I will briefly tell you the highlights of this fun and interesting project.
Why engagement?
Over the last years, the concept engagement has been of increasing interest within multiple domains: organization behavior, marketing, social psychology, and education [1]. For example marketing is very interested in engaged consumers, especially since research has shown that highly engaged consumers spend 60% more in each transaction, make 90% more frequent purchases, and are four times more likely to advocate for the brand [2]. No wonder companies are extremely interested in finding ways to increase their consumers’ level of engagement.
What does an engaged consumer look like?
But how do you know a consumer is feeling (highly) engaged? What does an engaged consumer look like? Well, for example, to test if advertisements are increasing one’s level of engagement, you can look at the facial expressions people show while watching the advertisements.
We all know that people have a hard time hiding their real feelings. Even back in 1872, Darwin already noticed that there is a strong link between experienced emotions and facial expressions [3].
To measure facial expressions, an automated facial expression analysis tool like FaceReader can be used. Aside from accurately measuring the basic emotions, FaceReader has the option to create custom expressions, where you can design your own algorithm by combining variables such as facial expressions and Action Units (AUs).
FREE WHITE PAPER: Facial Action Coding System
Download here the FREE white paper 'Facial Action Coding System (FACS)' and learn more about:
- Objective results with FACS
- Action Units
- Examples using FACS
What is engagement?
So what exactly is engagement? It’s a broad concept with lots of room for interpretation. A thorough literature review learned that many articles have been addressing the concept engagement, all with fairly overlapping but yet slightly different definitions.
What emerges was that engagement can be categorized into three components, namely emotional, cognitive, and behavioral engagement, as you can see below in Figure 1.
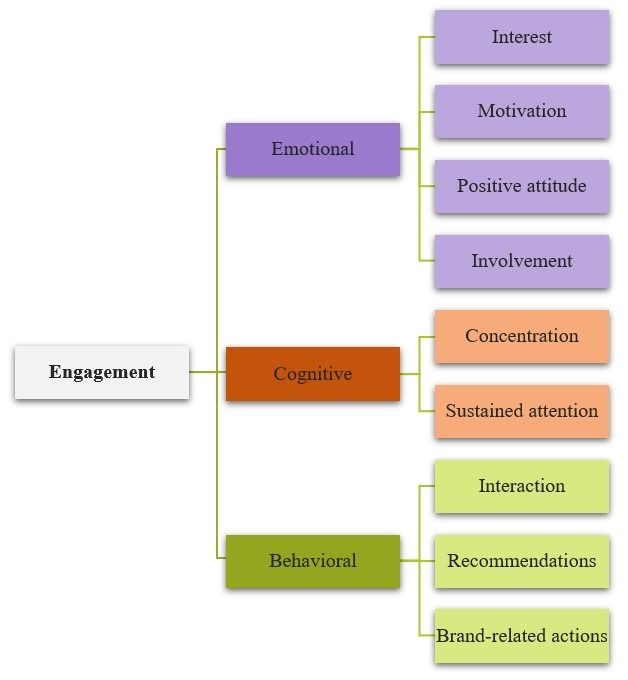
Figure 1. Hierarchy of engagement according to literature [4, 5, 6, 7, 8, 9].
With the information from literature, I’ve created a definition for engagement:
“Engagement seems to be the product of a two-way interaction, initiated by either the individual or the organization. On the one hand, the organization has to attract someone’s attention by presenting themselves as something that is appealing to the individual. They need to hold that attention long enough for a person to provoke a second level of attention, where feelings of involvement, interest, and motivation seem to contribute to this sustained attention. On the other hand arises the individuals’ free will to deliberately connect with the organization on such a level that a person is willing to go beyond the personal requirements associated with the organization.”
Now that the definition was made, it was time to gather information to create an algorithm.
The expressions of an engaged face
What does an ‘Engaged person’ look like? In literature, I found lots of contradictory information. From lots of expression versus no expression at all, to negative and positive correlations of expressions with engagement.
I decided to do my own experiment and found out myself what an engaged face looks like. For this purpose, I’ve created an online survey with the use of Qualtrics and FaceReader Online, where the stimuli (movie trailers) were set up in FaceReader Online and later embedded in Qualtrics with JavaScript.
Movie trailers were specifically chosen as stimuli because their duration is typically two to three minutes, which is long enough to evoke sustained attention, yet remains within the limits (0-3 minutes) of losing this sustained attention [10]. Thereby, movie trailers are specially designed to attract and maintain someone’s attention [11]. These movies were not out in theatres yet, so no bias could influence the experience.
People were invited to participate in a Qualtrics survey, and were recorded with their own webcam while looking at movie trailers, after which they were asked to rate their overall feelings of engagement per movie trailer. The recordings were exported to FaceReader 8.1 for extensive analyses, and the survey answers could be exported from Qualtrics to Excel and SPSS. Amazing how well all programs worked together!
FREE WHITE PAPER: FaceReader methodology
Download the free FaceReader methodology note to learn more about facial expression analysis theory.
- How FaceReader works
- More about the calibration
- Insight in quality of analysis & output
To analyze the results, I used the self-reported scores of level of overall engagement to create two groups: High Engaged people (scoring 70 and higher) and Low Engaged people (scoring 30 and lower). I analyzed both groups in FaceReader 8.1, and calculated the mean activation of expressions and AUs per participant in Excel.
With the AUs, only the intensity of AU 4 (Brow Lowerer) was slightly higher with Low Engaged people. As you can see in de bar chart below (Figure 2), the difference between the expressions of High and Low Engaged people was also very small. It is interesting to see that High Engaged people score higher on Neutral, which was quite unexpected.
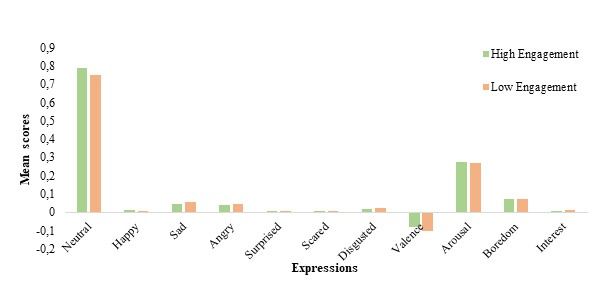
Figure 2. A bar chart visualizing and comparing the mean results of all the participants on the seven expressions (basic emotions), two custom expressions (boredom and interest) and valence & arousal.
Validation study
So unfortunately, my experiment did not help me much with creating an algorithm. Therefore, I decided to create not just one but two custom expressions, based on both the information from literature and the results from my first experiment.
The condition was set that there can be no engagement with a high boredom score. With a low boredom score, the Valence score is taken. Valence is chosen because a Valence score of 0 is equal to a high Neutral score, exactly the main results from my first experiment. With one custom expression, I subtracted AU 4 from the Valence score, since my first experiment showed that AU 4 was slightly higher with Low Engaged people.
To test these custom expressions, I have set up a second online experiment, again with Qualtrics and FaceReader Online. This time I used advertisements for people to look at, so I could record their expressions. Afterwards, they were asked to rate their overall feelings of engagement, so I could compare the activation score of the custom expressions with the self-reported engagement scores. Unfortunately, there was no significant effect, meaning that the custom expressions could not be validated.
Despite this disappointing result, some interesting findings need to be mentioned when looking at an individual level. Figure 3 shows that the custom expressions of some participants are on the right track. This can be used as a starting point for follow-up research.
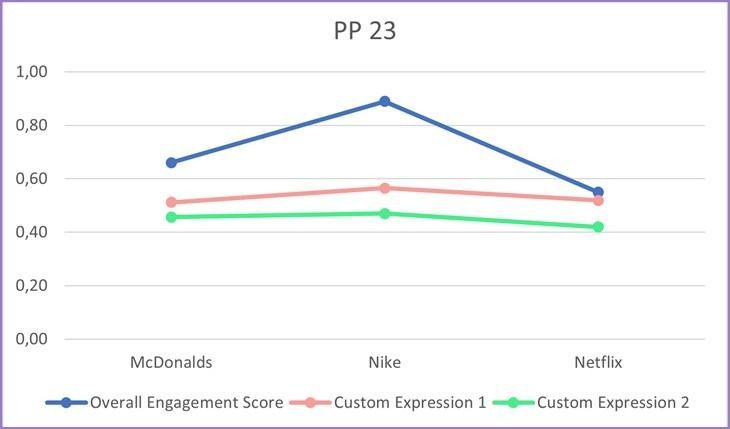
Figure 3. Scores of participants 23
Recommendations
First and foremost, I would highly recommend to look at engagement as an affective state which is prolonged in nature, and should be measured as an overall score, rather than an expression being measured and estimated per frame, such as basic expressions like happy or angry.
Also, for follow-up research it might be interesting to measure engagement in groups or pairs, especially if you keep in mind that the expression of emotions is a form of communication. When looking at stimuli as a couple, you might show more expression.
Lastly, due to COVID-19, I had to execute all experiments online. The advantage was that I could reach more participants, since they could participate from home. In this way the COVID-19 regulations could be respected.
Yet the disadvantage was that, when using the participants’ webcam instead of the Noldus lab camera, several analyses like heart rate and head orientation could not be used, and we had less control over the quality of the recordings compared to a lab study. Using the Noldus Experience Lab for follow-up research might gain some interesting findings!
Despite the fact that the custom expressions for engagement could not yet be validated, this research showed insights in the concept of engagement. When keeping the recommendations in mind, follow-up research is highly recommended. Interested in using FaceReader for follow-up research? Contact Noldus for more information!
If you are interested in continuing this validation study with use of FaceReader data, please contact Niek Wilmink for more information.
References
- Alvarez-Milán, A., Felix, R., Rauschnabel, P. A., & Hinsch, C. (2018). Strategic customer engagement marketing: A decision making framework. Journal of Business Research, 92, 61-70. https://doi.org/10.1016/j.jbusres.2018.07.017
- Rosetta Consulting (2014). The economics of engagement (pp. 1–9). Retrieved from http://www.rosetta.com/assets/pdf/The-Economics-of-Engagement.pdf.
- Wallbott, H. G. (1998). Bodily expression of emotion. European journal of social psychology, 28(6), 879-896.
- Christenson, S. L., Reschly, A. L., & Wylie, C. (Eds.). (2012). Handbook of research on student engagement. Springer Science & Business Media
- Trowler, V. (2010). Student engagement literature review. The higher education academy, 11(1), 1-15.
- Harmeling, C. M., Moffett, J. W., Arnold, M. J., & Carlson, B. D. (2017). Toward a theory of customer engagement marketing. Journal of the Academy of marketing science, 45(3), 312-335.
- Brodie, R. J., Hollebeek, L. D., Jurić, B., & Ilić, A. (2011). Customer engagement: Conceptual domain, fundamental propositions, and implications for research. Journal of service research, 14(3), 252-271. https://doi.org/10.1177/1094670511411703
- Hollebeek, L. D., Jurić, B., & Ilić, A. (2011). Customer engagement: Conceptual domain, fundamental propositions, and implications for research. Journal of service research, 14(3), 252-271.
- O’Brien, H., & Cairns, P. (2015). An empirical evaluation of the User Engagement Scale (UES) in online news environments. Information Processing & Management, 51(4), 413-427. https://doi.org/10.1016/j.ipm.2015.03.003
- Guo, P. J., Kim, J., & Rubin, R. (2014). How video production affects student engagement: An empirical study of MOOC videos. In Proceedings of the first ACM conference on Learning@ scale conference, 41-50. https://doi.org/10.1145/2556325.2566239
- Wang, L., Liu, D., Puri, R., & Metaxas, D. N. (2020). Learning trailer moments in full-length movies with co-contrastive attention. arXiv preprint arXiv:2008.08502.
Related Posts
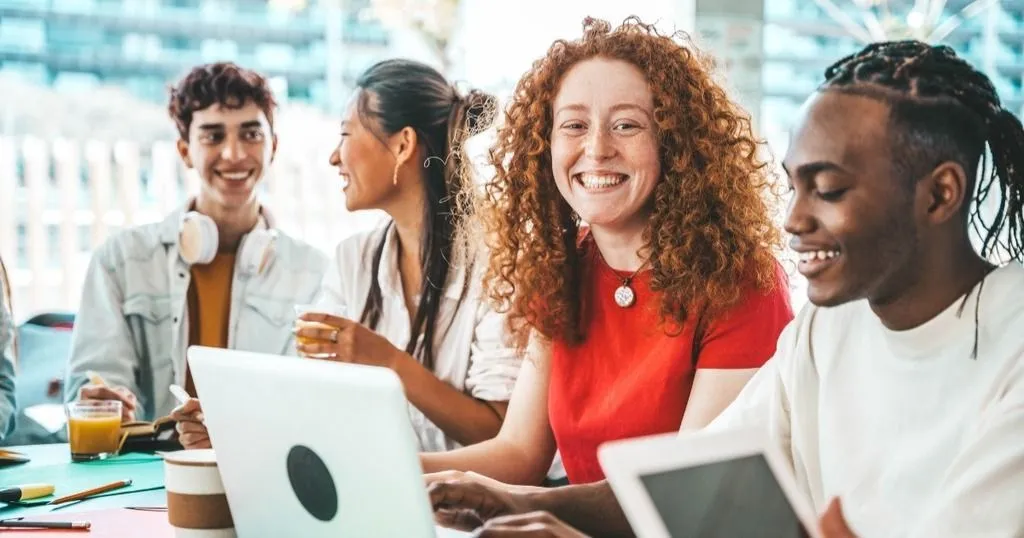
How to increase attention in commercials for Gen-Z
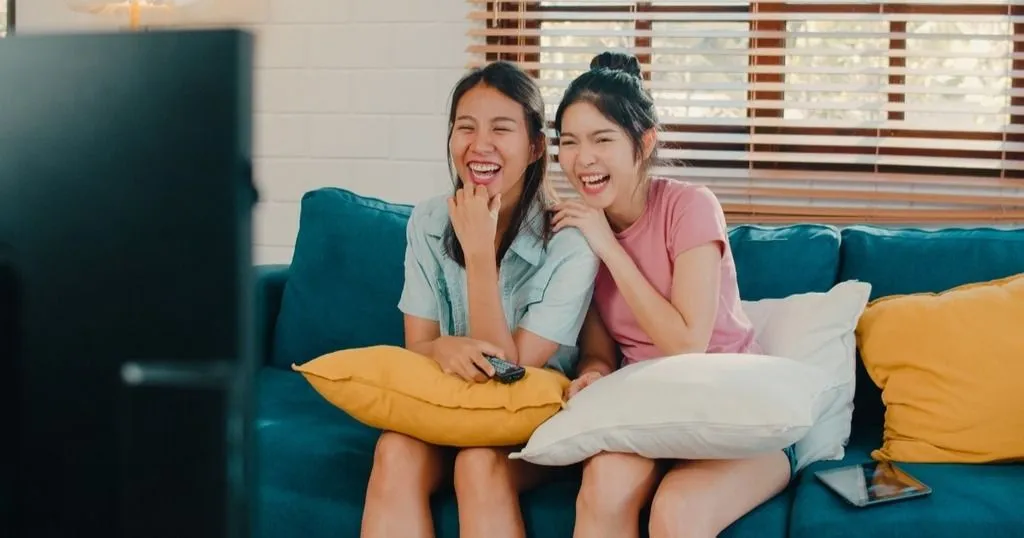
How does perception of brand authenticity affect ad performance?
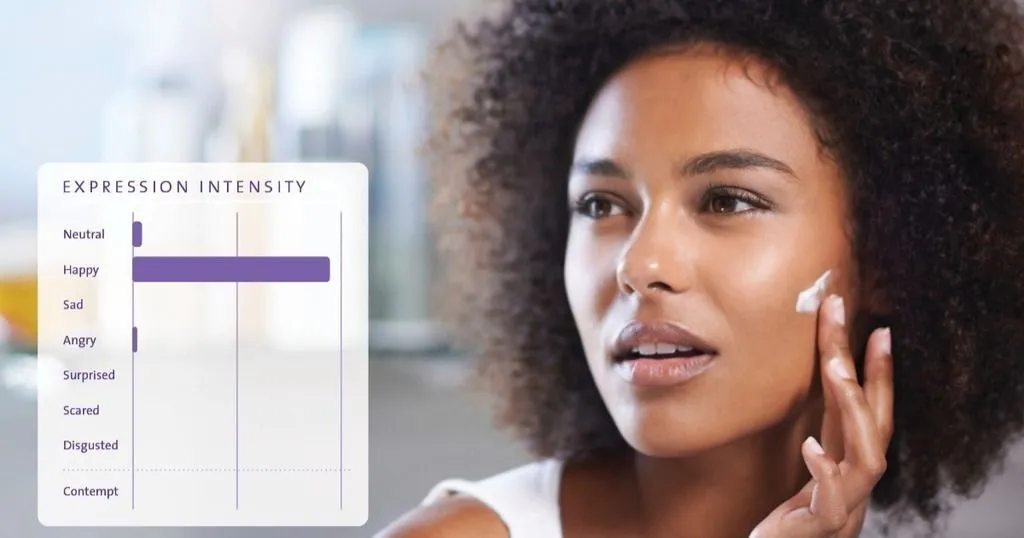