8 reasons why FaceReader outperforms OpenFace
In this blog post, we’ll break down why FaceReader outperforms OpenFace. We’ll focus on key aspects like accuracy, ease of use, and security—making sure you get reliable results without the hassle.
Posted by
Published on
Mon 17 Feb. 2025
Topics
| FaceReader | Facial Expression Analysis | FACS | Emotions | Measure Emotions | Behavioral Research |
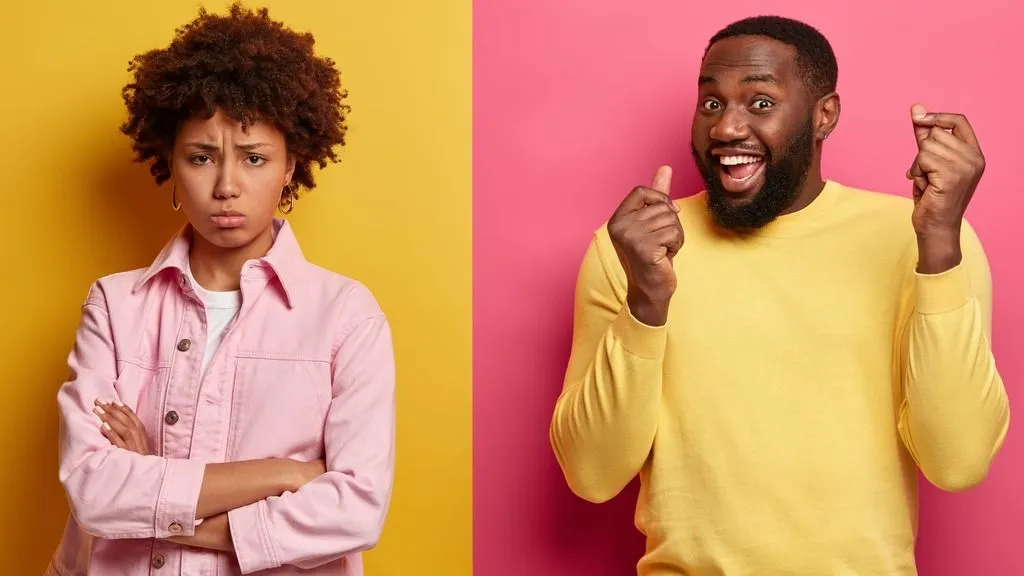
Facial expression analysis can reveal a lot about human emotions, making it a powerful tool in research. But with so many options out there, how do you choose the right software?
In this blog post, we’ll break down why FaceReader outperforms OpenFace. We’ll focus on key aspects like accuracy, ease of use, and security—making sure you get reliable results without the hassle. Keep reading to see why FaceReader is the top choice for your facial expression analysis.
Why should you measure facial expressions?
Emotions influence our behavior—from deciding what to eat and whether to buy a product to our interactions with others. That's why researchers in psychology, user experience, neuroscience, and more include emotion analysis in their studies.
One way to measure emotions is to ask people about them. However, often we're not fully aware of our emotions in the moment, or don't know how to describe them accurately. If you want to know more about people's unconscious and immediate reactions, facial expression analysis is the way to go.
Objective facial expression analysis for your research
FaceReader is the most robust tool for automated facial expression analysis there is. It's easy to use, gives you reliable data, and saves you a lot of time. Plus, it provides many options to visualize and analyze your data, making it easier to interpret your results.
And there's more to FaceReader than determining the 6 basic expressions—happy, sad, angry, surprised, scared, and disgusted. The software also measures the smallest visible movements in people's facial muscles, which are called Action Units (AUs). These AUs are based on the widely used Facial Action Coding System (FACS).
RESOURCES: Read more about FaceReader
Find out how FaceReader is used in a wide range of studies and how it can elevate your research!
- Free white papers
- Customer success stories
- Featured blog posts
Automated analysis is objective and saves time
Classifying these AUs by hand is considered the gold standard in research—as long as you're a FACS certified coder. This means getting at least a 70% accuracy score on the FACS Final Test. But that requires extensive training and is very time-consuming.
And even when you have a FACS coder on your team, processing hours of facial recordings can take days or even weeks. Automated analysis tools like FaceReader are a great way to get objective data—fast.
Dr. Erin Heerey uses FaceReader in the Social Behavior Lab at Western University. Frame-by-frame expression analysis of her project would have taken 800 hours of manual coding. FaceReader did it in only 14 hours!
8 reasons why FaceReader is better than OpenFace
When choosing the right tool for your facial expression analysis, it's important to consider accuracy, reliability, and ease of use. And, of course, you need guarantees that your data will be safe.
In this blog post, we compare FaceReader's performance with that of OpenFace, an open-source tool for automated facial expression analysis. Here's why FaceReader is the superior choice compared to OpenFace:
1. FaceReader meets FACS agreement—OpenFace does not
A comparison by VicarVision clearly shows that FaceReader has higher precision than OpenFace. With an FACS agreement score of 0.811 and 0.792, FaceReader more than meets the threshold of the internationally agreed FACS Final Test (0.70). This ensures that your data are reliable and valid. OpenFace, however, does not meet these minimal requirements, with an agreement index of 0.621 and 0.672.
Think of it like trying to drive a car without a license—OpenFace simply doesn't provide the precision needed for high-quality research. With FaceReader, you can trust that you get robust and accurate research outcomes.
2. FaceReader is ready to use immediately
When you buy FaceReader, it is already configured, allowing you to start analyzing facial expressions right out of the box. It’s designed with a user-friendly interface, so you don’t have to waste time setting up or programming.
OpenFace, on the other hand, requires significant effort to configure and program. For researchers, especially those in user experience or psychology, FaceReader’s ease of use means you can get started right away with your research.
Specifically, FaceReader also includes real-time emotion classification and detection of custom expressions. OpenFace lacks these features and requires time-consuming custom scripting.
3. OpenFace works with outdated models
FaceReader’s models are powered by deep learning and AI. They are also regularly updated, with the latest innovations incorporated. This ensures that you're always working with the most accurate models for facial expression analysis.
In contrast, OpenFace relies on models that are over six years old. The software has only released two major versions, with the latest (v2.2) dating back to 2018/2019. As a result, many of the models OpenFace uses have become outdated, which can affect the accuracy of your facial expression analysis.
This outdated technology can be especially problematic when working in real-world conditions, where you may encounter varying lighting or movement of participants.
4. FaceReader is more precise—with 468 landmarks versus OpenFace's 67
OpenFace only uses 67 landmarks in their face models, while FaceReader relies on 468. This allows FaceReader to provide a far more detailed and accurate analysis of facial expressions, with better precision. With fewer landmarks, OpenFace can only provide a rough estimate for some facial features, which reduces accuracy.
FaceReader’s higher landmark count improves image pre-processing for facial analysis, which strengthens face detection and modeling. Again, this benefits researchers working in challenging conditions like low lighting or high movement.
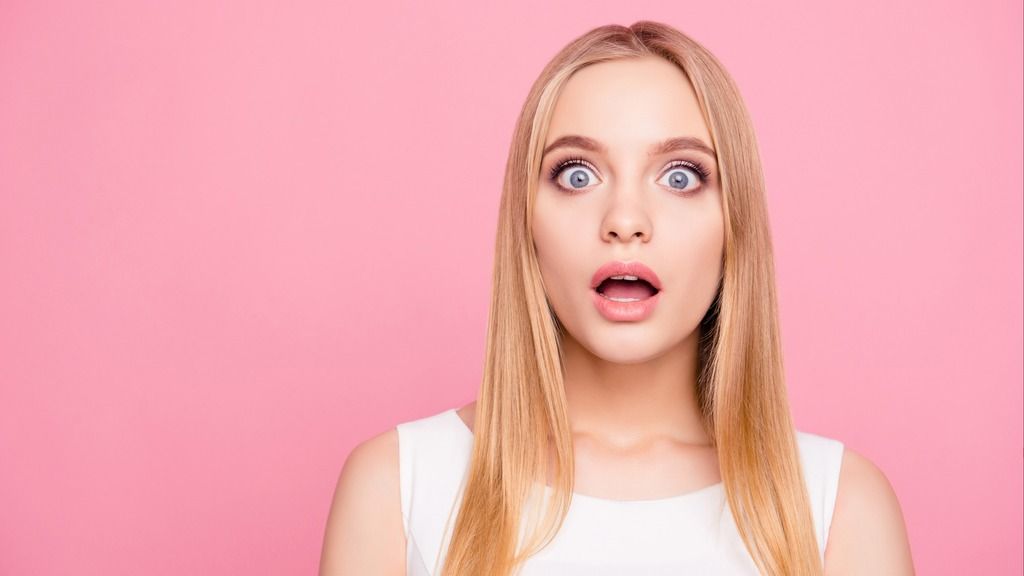
5. Better analysis and visualizations with FaceReader
With FaceReader, you can analyze and visualize data in different ways. This helps you gain better insights into emotional responses.
For example, you'll find emotion classifications, information about gaze or head orientation, and a chart showing levels of valence and arousal. You can also combine your FaceReader analysis with eye tracking, enriching your data even further.
OpenFace, while capable of processing data, offers minimal options to visualize and interpret your results or expand your setup. For example, you won't even find emotion classifications within the software, leaving you to deal with basic data. OpenFace also includes fewer Action Units than FaceReader.
6. FaceReader incorporates the latest techniques
FaceReader is continuously evolving, incorporating new techniques and features in every update. This includes options like measuring heart rate and heart rate variability (HRV), creating your own custom expressions, and observing Action Units on the left and right side of the face separately.
Moreover, FaceReader Online (FRO) allows for remote data collection, combined with integrated survey tools. This way, you can study participants in their natural environments, from anywhere you want.
In contrast, OpenFace has had minimal updates since 2018, and it doesn’t offer any guarantees about future improvements due to its open-source nature.
FREE WHITE PAPER: FaceReader custom expressions
Download this free white paper to learn more about how to create custom expressions in FaceReader.
- How to combine different metrics
- Best practices for custom expressions
- Examples from other researchers
7. FaceReader has better data privacy and security than open-source tools
When it comes to data privacy, FaceReader offers a more secure and reliable solution. FaceReader is fully GDPR compliant, with centralized, encrypted storage. This ensures that all data is protected and secure.
This level of protection isn’t guaranteed with OpenFace’s open-source system, which can expose your data to security risks. With FaceReader, you can be confident that sensitive data is not only protected, but also managed in a way that meets the highest privacy standards.
8. Always get the help you need with excellent customer support from Noldus
At Noldus, you benefit from exceptional customer support. From installation to ongoing training, we offer personalized, expert assistance to ensure your research runs smoothly. Our team includes researchers with real-world experience, which means you’re getting advice from people who understand your research needs.
OpenFace, however, lacks dedicated support and only offers assistance through a general forum. This can be frustrating when you run into technical issues.
FaceReader: the reliable, efficient choice for facial expression analysis
In short, FaceReader is the top choice for researchers who need precise and efficient facial expression analysis. The software's ability to match the accuracy levels of FACS coders ensures that the data you collect are reliable and scientifically valid.
FaceReader's user-friendly interface, fast processing speed, and built-in privacy measures make it an ideal tool for researchers who want to focus on their studies without the hassle of programming or complex setups.
Whether you're in psychology, user experience, or neuroscience—FaceReader offers the setup you need to take your research to the next level.
References
- Compared to classifications of the Amsterdam Dynamic Facial Expression Set (ADFES) dataset
Van der Schalk, J.; Hawk, S.; Fischer, A.; Doosje, B. (2011). Moving faces, looking places: Validation of the Amsterdam Dynamic Facial Expression Set (ADFES). Emotion, 11(4), 907–920. https://doi.org/10.1037/a0023853
- Compared to classifications of the Warsaw Set of Emotional Facial Expression Pictures (WSEFEP) dataset.
Olszanowski, M.; Pochwatko, G.; Kuklinski, K.; Scibor-Rylski, M.; Lewinski, P.; Ohme, R. (2014). Warsaw set of emotional facial expression pictures: a validation study of facial display photographs. Frontiers in Psychology, 5. https://doi.org/10.3389/fpsyg.2014.01516
Related Posts
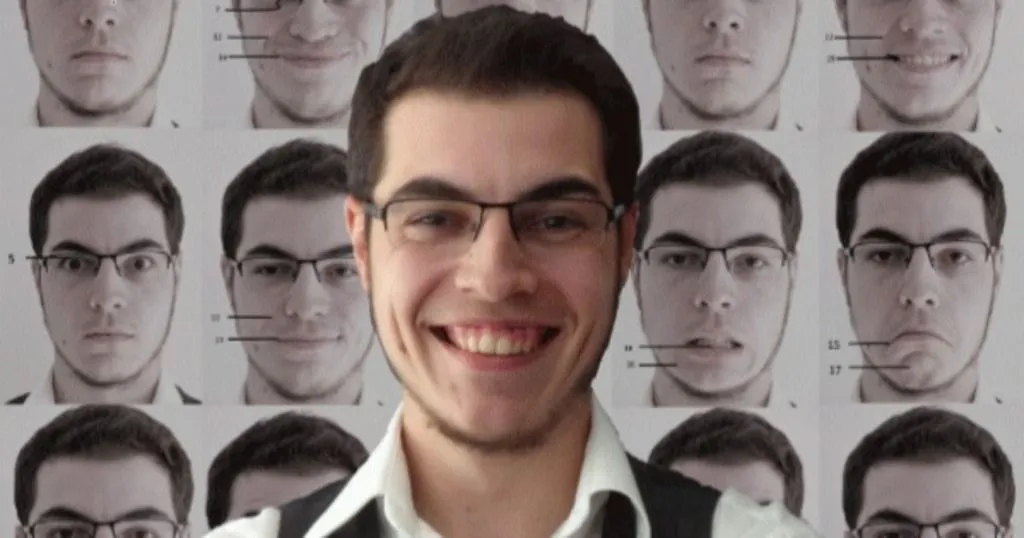
Validation-study: Basic emotions and Action Units detection
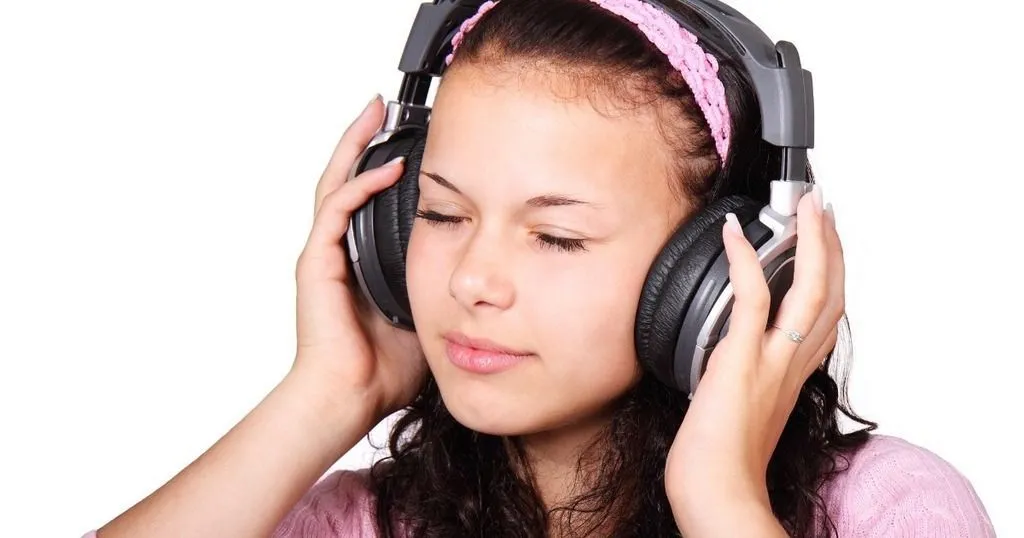
Using facial expression analysis during a musical experiment
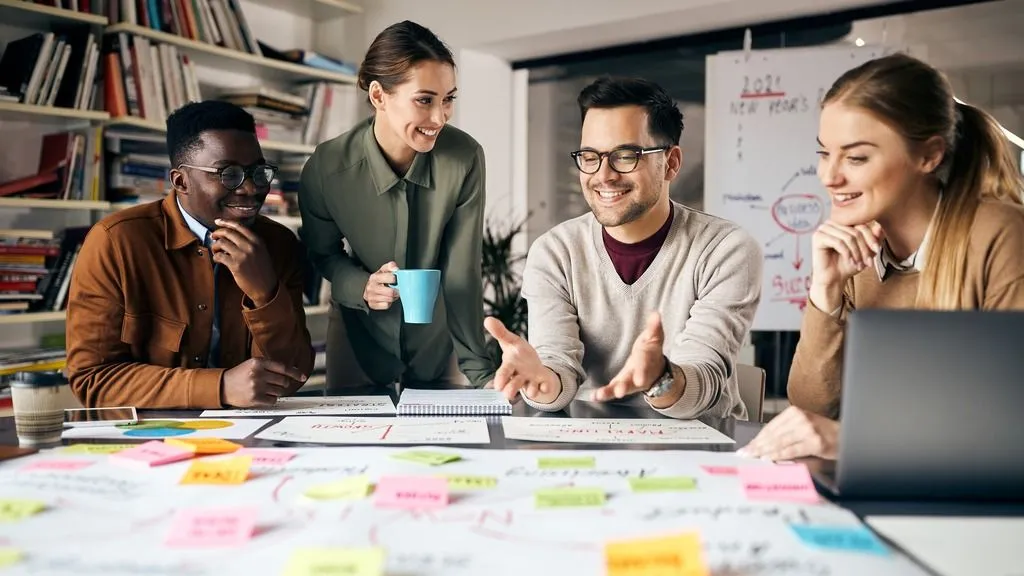